Review of Predictions of Impact Performances and Damages of Fiber Reinforced Composite using Machine Learning Approaches
DOI:
https://doi.org/10.37934/mjcsm.16.1.274315Keywords:
Fiber-reinforced composite, impact performance and damage, machine learningAbstract
Fiber-reinforced composites (FRCs) offer high specific mechanical properties like-exceptional strength, lightweight properties, and versatility, but their susceptibility to impact damage poses a significant challenge. Characterizing these while connecting processing methods, microstructure, and environmental factors to impact response has seen limited success through conventional modeling. But with increasing computational power and availability of data, machine learning techniques present opportunities in this domain enabling accurate prediction and robust monitoring of impact performance and damage. This review paper provides a comprehensive examination of the evolving landscape in predicting impact performances and damages of FRCs to optimize composites’ design through the lens of different supervised, unsupervised, blended, deep transfer learning and alternative approaches highlighting their strengths, limitations, and suitability for specific tasks. Methods encompassing artificial neural networks (ANNs), support vector machines (SVMs), and convolutional neural networks (CNNs) have exhibited promise in predicting performance and damage parameters respectively. Each section critically evaluates the strengths, limitations, and contributions of these approaches, providing a holistic view of their effectiveness.Downloads
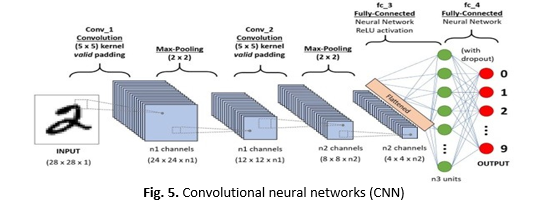