Carbon Reduction Analysis of Malaysian Green Port Operation
Keywords:
Carbon Reduction, Malaysian Ports, Green Port Operation, Forecasting AnalysisAbstract
With the recently adopted carbon policy in Malaysia to reduce carbon emissions by 45% on the year 2030, this paper analyses the carbon reduction efforts from the port sector. Driven by strong trade growth that fuels the transshipment volume, significant emission is produced from major ports. Therefore, the aim of this project is to forecast the emissions produced by ports accounting for continuous growth. Then, the projected emission will be compared and analysed to the levels of reduction committed by Malaysia national policy. After analysing the time-series TEU trend, ARIMA forecasting technique was used to forecast the carbon emissions performance by initiatives of LED conversion, adoption of E-RTG, and E-PM. The results indicated overall carbon emission growth at an average of 5.73% per year. No reduction in both direct (2.80% per year) and indirect carbon emission (5.91% per year), which leaves the targets of Malaysian Carbon policy unmet. A more robust blueprint of carbon emissions reduction map is recommended with the main component of electrifying the prime mover fleet.
References
C. Le Quéré, R.M. Andrew, P. Friedlingstein, S. Sitch, J. Hauck, et al. Global Carbon Budget 2018, Earth System Science Data 10 (2018) 2141–2194. https://doi.org/10.5194/essd-10-2141-2018.
The Kyoto Protocol: Climate Change Success or Global Warming Failure? - Circular Ecology, Circular Ecology, 2015. https://circularecology.com/news/the-kyoto-protocol-climate-change-success-or-global-warming-failure.
L. Yang, Y. Cai, X. Zhong, Y. Shi, Z. Zhang, A Carbon Emission Evaluation for an Integrated Logistics System—A Case Study of the Port of Shenzhen, Sustainability. 9 (2017) 462. https://doi.org/10.3390/su9030462.
Anne-Marie Causer, SAFETY AND ENVIRONMENTAL MANAGEMENT CERTIFICATION, 2008. https://www.greenport.com/news101/Regulation-and-Policy/safety-and-environmental-management-certification (accessed 13 March 2020).
E. Lawer, J. Herbeck, M. Flitner, Selective Adoption: How Port Authorities in Europe and West Africa Engage with the Globalizing ‘Green Port’ Idea. Sustainability 11 (18) (2019) 5119. https://doi.org/10.3390/su11185119.
G.E. Box, G.M. Jenkins, G.C. Reinsel, G.M. Ljung, Time series analysis: forecasting and control, John Wiley & Sons, 2015.
G. Zhang, B. Eddy Patuwo, M. Y. Hu, Forecasting with artificial neural networks: The state of art, International Journal of Forecasting 14 (1998) 35-62. https://doi.org/10.1016/s0169-2070(97)00044-7.
P. Firmino, P. de Mattos Neto, T. Ferreira, Correcting and combining time series forecasters, Neural Networks 50 (2014) 1-11. https://doi.org/10.1016/j.neunet.2013.10.008.
N. Kourentzes, D. Barrow, S. Crone, Neural network ensemble operators for time series forecasting, Expert Systems with Applications. 41 (2014) 4235-4244. https://doi.org/10.1016/j.eswa.2013.12.011.
D. Ömer Faruk, A hybrid neural network and ARIMA model for water quality time series prediction, Engineering Applications of Artificial Intelligence 23 (2010) 586-594. https://doi.org/10.1016/j.engappai.2009.09.015.
T. Koutroumanidis, K. Ioannou, G. Arabatzis, Predicting fuelwood prices in Greece with the use of ARIMA models, artificial neural networks and a hybrid ARIMA–ANN model, Energy Policy 37 (2009) 3627-3634. https://doi.org/10.1016/j.enpol.2009.04.024.
G. Zhang, Time series forecasting using a hybrid ARIMA and neural network model, Neurocomputing 50 (2003) 159-175. https://doi.org/10.1016/s0925-2312(01)00702-0.
P. Firmino, P. de Mattos Neto, T. Ferreira, Error modeling approach to improve time series forecasters, Neurocomputing 153 (2015) 242-254. https://doi.org/10.1016/j.neucom.2014.11.030.
M. Khashei, M. Bijari, An artificial neural network (p,d,q) model for timeseries forecasting, Expert Systems with Applications. 37 (2010) 479-489. https://doi.org/10.1016/j.eswa.2009.05.044.
M. Khashei, M. Bijari, A novel hybridization of artificial neural networks and ARIMA models for time series forecasting, Applied Soft Computing. 11 (2011) 2664-2675. https://doi.org/10.1016/j.asoc.2010.10.015.
J. Stouch, Lighting Comparison: LED versus HID Lights, Stouchlighting.Com., 2015. https://www.stouchlighting.com/blog/lighting-comparison-led-versus-hid (accessed 13 April 2020).
Average CO2 emissions from new cars and new vans increased in 2018, European Environment Agency, EEA., 2019. https://www.eea.europa.eu/highlights/average-co2-emissions-from-new (accessed 20 April 2020).
Konecranes, Power Options for RTGs, 2012. https://www.konecranes.com/sites/default/files/download/konecranes_power_options_brochure_final.pdf (accessed 15 April 2020).
Kalmar, Kalmar Zero Emission RTG, 2019. https://www.kalmarglobal.com/equipment-services/rtg-cranes/zero-emission/ (accessed 3 May 2020)
Deauto Industry, Specifications of CAMC Prime Mover, 2014. https://www.deautoind.com.my/prime.html (accessed 3 May 2020).
MOT, Stastic of Maritime Transport, 2008. http://www.mot.gov.my/en/resources/maritime-statistic (accessed 23 April 2020).
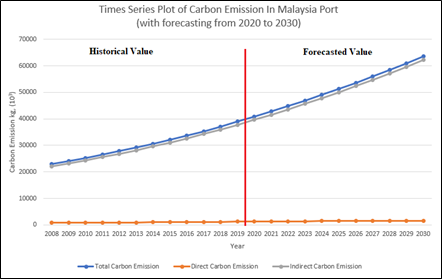
Downloads
Published
How to Cite
Issue
Section
License
Copyright (c) 2021 Progress in Energy and Environment

This work is licensed under a Creative Commons Attribution-NonCommercial 4.0 International License.